Why Supervised Learning Works?
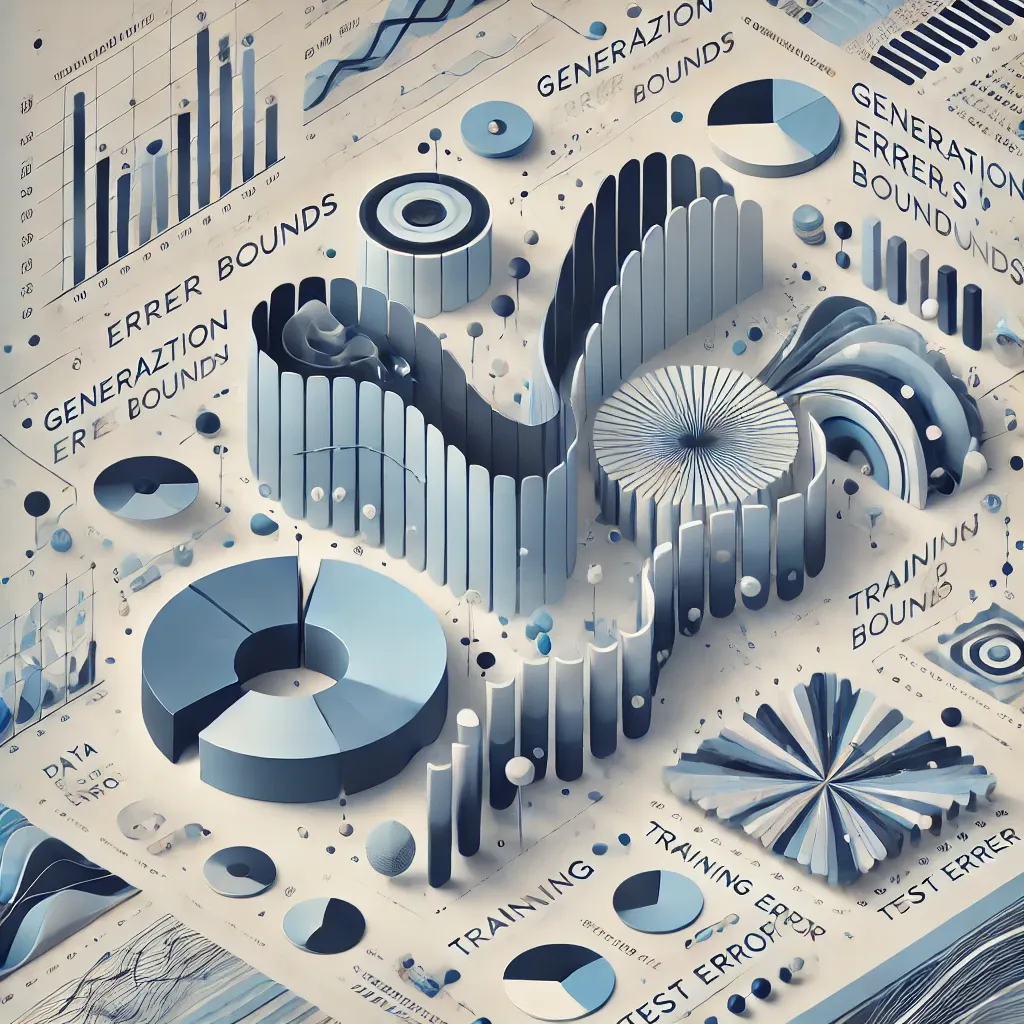
Machine learning algorithms, such as logistic regression and XGBoost, are widely used in various industries. Practitioners typically focus on whether a technique is effective without a strong interest in understanding the reasons behind its success or failure. They may adopt a brute-force approach to identify the best features and algorithms for their out-of-time validation. In this blog, I will explain why supervised learning works. The statistical learning theory is not my original idea, but I hope that by sharing it with a wider audience, I can contribute to the distribution of knowledge.
In essence, "Low Training Error + Sufficient Data Relative to Model Complexity → Low Test Error".
Supervised learning must work on the SAME distribution.
Key Concepts
- Training Error (\(\text{Train}_S(f)\)): The error of a model \(f\) evaluated on the training dataset \(S\).
- Test Error (\(\text{Test}_D(f)\)): The expected error of \(f\) on new, unseen data drawn from the same distribution \(D\).
- Hypothesis Class (\(\mathcal{F}\)): The set of all models \(f\) that the learning algorithm can choose from.
- Sample Size (\(|S|\)): The number of training examples.
- Confidence Level (\(1 - \delta\)): The probability that the bound holds true.
- Degrees of Freedom: Informally, the number of parameters or complexity of the model class \(\mathcal{F}\).
Mathematical Formulation
Generalization Error Bound
The generalization error bound is given by:
\[ \Pr_{S \sim D^{|S|}} \left[ \text{Test}_D(f) - \text{Train}_S(f) \leq \sqrt{\frac{\log |\mathcal{F}| + \log \frac{1}{\delta}}{|S|}} \quad \text{for all} \quad f \in \mathcal{F} \right] > 1 - \delta \]
Interpretation:
With probability at least \(1 - \delta\), the difference between test and training error is bounded by:
\[ \sqrt{\frac{\log |\mathcal{F}| + \log \frac{1}{\delta}}{|S|}} \]
Implications:
- Sample Size Effect
- Larger training sample size \(|S|\) results in:
- Tighter error bounds
- Test error closer to training error
- Larger training sample size \(|S|\) results in:
- Model Complexity Effect
- Larger hypothesis class size \(|\mathcal{F}|\) leads to:
- Looser error bounds
- Potentially larger gap between test and training errors
- Larger hypothesis class size \(|\mathcal{F}|\) leads to:
Proof
The proof follows these key steps:
- Setup
- Let \(f \in \mathcal{F}\) be any hypothesis
- Let \(S\) be a training set of size \(|S|\) drawn i.i.d. from distribution \(D\)
- Let \(\text{Test}_D(f)\) and \(\text{Train}_S(f)\) be the test and training errors
- Hoeffding's Inequality
For a single hypothesis \(f\):
\[ \Pr_S[|\text{Test}_D(f) - \text{Train}_S(f)| > \epsilon] \leq 2e^{-2|S|\epsilon^2} \]
Note on Hoeffding's Inequality: In probability theory, Hoeffding's inequality provides an upper bound on the probability that the sum of independent random variables deviates from its expected value.
- Union Bound
Apply to all hypotheses \(f \in \mathcal{F}\):
\[ \Pr_S[\exists f \in \mathcal{F}: |\text{Test}_D(f) - \text{Train}_S(f)| > \epsilon] \leq |\mathcal{F}| \cdot 2e^{-2|S|\epsilon^2} \]
- Set Failure Probability
Let right side equal \(\delta\):
\[ |\mathcal{F}| \cdot 2e^{-2|S|\epsilon^2} = \delta \]
- Solve for \(\epsilon\)
\[ \begin{align*} e^{-2|S|\epsilon^2} &= \frac{\delta}{2|\mathcal{F}|} \\ -2|S|\epsilon^2 &= \ln(\frac{\delta}{2|\mathcal{F}|}) \\ \epsilon^2 &= \frac{\ln(2|\mathcal{F}|/\delta)}{2|S|} \\ \epsilon &= \sqrt{\frac{\ln(2|\mathcal{F}|/\delta)}{2|S|}} \end{align*} \]
- Final Result
Since \(\Pr_S[\exists f \in \mathcal{F}: |\text{Test}_D(f) - \text{Train}_S(f)| > \epsilon] \leq \delta\), it follows that the probability that all hypotheses satisfy the inequality is at least \(1-\delta\): \(\Pr_S[\forall f \in \mathcal{F}: |\text{Test}_D(f) - \text{Train}_S(f)| \leq \epsilon] \geq 1 - \delta\).
Thus, with probability at least \(1-\delta\), the difference between test and training error is bounded by:
\[ |\text{Test}_D(f) - \text{Train}_S(f)| \leq \sqrt{\frac{\log |\mathcal{F}| + \log \frac{1}{\delta}}{|S|}} \]
This proves that with high probability, the difference between test and training error is bounded by a term that depends on the model complexity (\(|\mathcal{F}|\)) and training set size (\(|S|\)).
In other words, supervised learning must work on the SAME distribution for these bounds to hold.
Comments ()